Researchers at the National Institutes of Health (NIH), led by visting fellow Dakai Jin, developed a deep learning-based system to automatically inpaint and detect pulmonary nodules, which are round or oval-shaped growths in the lung.
“Deep learning has achieved significant recent successes. However, large amounts of training samples, which sufficiently cover the population diversity, are often necessary to produce high-quality results” the researchers wrote in their paper. “Unfortunately, data availability in the medical image domain, especially when pathologies are involved, is quite limited due to several reasons: significant image acquisition costs, protections on sensitive patient information, limited numbers of disease cases, difficulties in data labeling, and large variations in locations, scales, and appearance.”
Using NVIDIA Tesla GPUs and the cuDNN-accelerated Caffe deep learning framework, the team trained their 3D conditional generative adversarial network on over 1,000 lung nodules. Once trained, the system generated thousands of additional synthetic lung nodules with different shapes and appearances.
“How to generate effective and sufficient medical training samples with limited or no expert-intervention is always an open and challenging problem,” said Dakai Jin, the lead researcher on this paper and a visiting fellow at the NIH. “Yet, the advent of generative adversarial network (GAN) has made game-changing strides in simulating real images or data.”
Jin says that by using this synthetic training data, he and his team were able to significantly improve the performance of pathological lung segmentation.
“Our system provides a feasible way to help overcome difficulties in obtaining data for edge cases in medical images; and we demonstrate that GAN-synthetized data can improve training of a discriminative model, in this case for segmenting pathological lungs,” the team said. “Our CGAN approach can provide an effective and generic means to help overcome the dataset bottleneck commonly encountered within medical imaging.”
When compared to three other neural networks – the proposed CGAN produces the best results in dice scores, Hausdorff distances, and average surface distances. Dice scores improve from 0.964 to 0.989. The Hausdorff and average surface distance are reduced by 2.4 mm and 1.2 mm respectively. In terms of visual quality, the proposed network produces considerable improvements in segmentation mask quality, the researchers said.
The paper was published on ArXiv, and will be presented at the 21st International Conference on Medical Image Computing and Computer Assisted Intervention (MICCAI), September 16 – 20 in Granada, Spain.
Read more >
AI Automatically Detects Lung Abnormalities
Jun 12, 2018
Discuss (0)
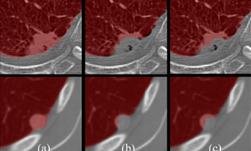
Related resources
- GTC session: Creating AI-Powered Hardware Solutions for Medical Imaging Applications
- GTC session: Reward Fine-Tuning for Faster and More Accurate Unsupervised Object Discovery
- GTC session: Early Diagnosis of Cancer Cachexia Using Body Composition Index as the Radiographic Biomarker
- NGC Containers: MONAI Toolkit
- SDK: MONAI Cloud API
- SDK: MONAI Deploy App SDK